The SAFEmig project
The project lays the ground for the theory of how a machine learns to take decisions independently. These decisions must be efficient concerning time, energy, and resources. At the same time, actions must be safe for people and the environment. There are important domains where the application of machine learning is still scarce in critical infrastructures such as autonomous vehicle, water supply and electricity grids, where safe operation is of foremost importance. Yet, ensuring safety with machine learning is an open challenge.
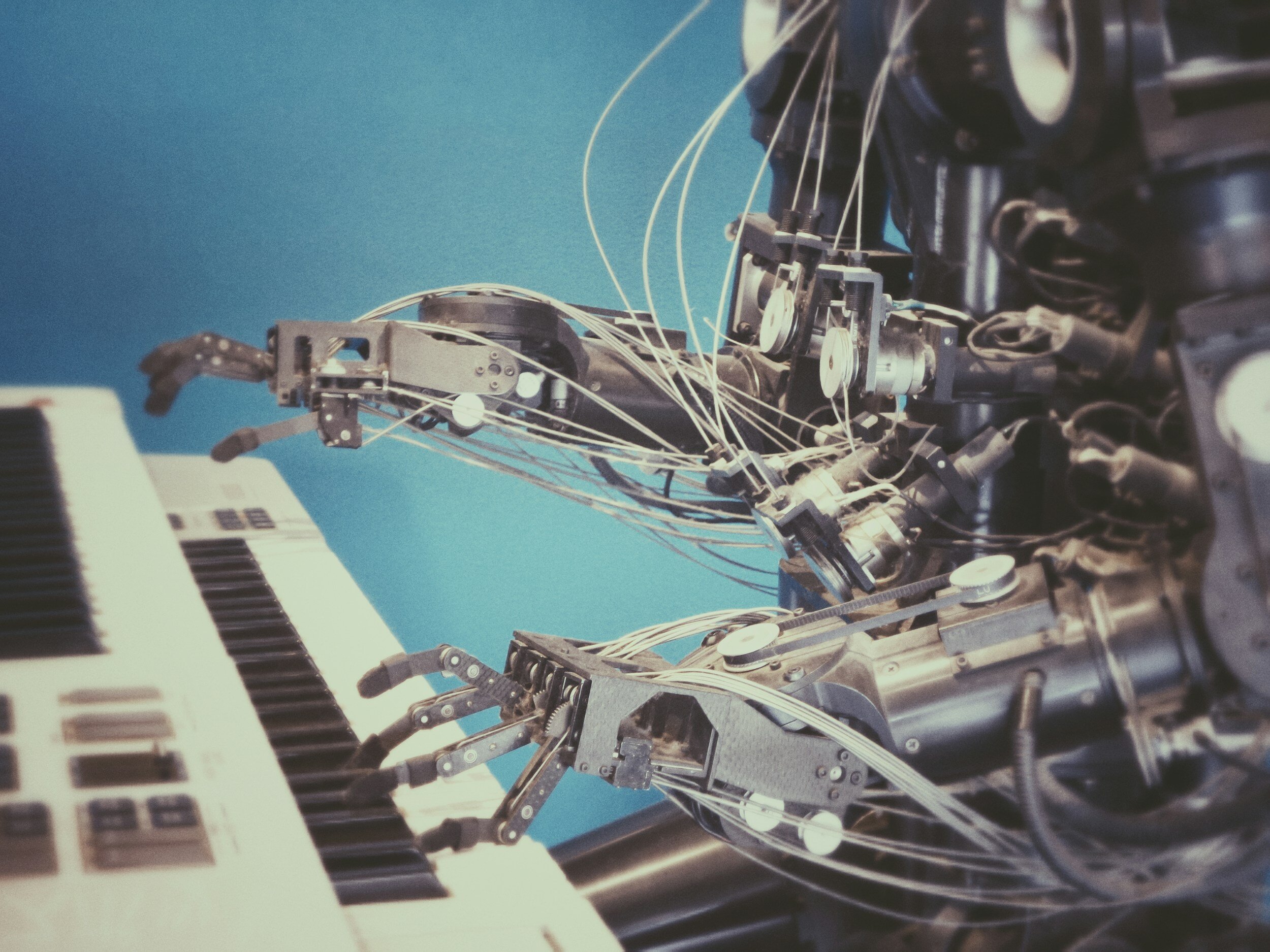
Have a chat with
ABHIJIT MAZUMDAR
About:
Safe Reinforcement Learning for Safety-Critical Systems
Maintaining safety is crucial during the operation of safety-critical systems. While reinforcement learning solves optimal control problems in a model-free setup, it does not ensure safety during the learning phase. Safe reinforcement learning circumvents this problem by embedding safety constraints into the learning process. Unlike conventional reinforcement learning, it uses safe actions more frequently during the exploration or learning phase. This method guarantees the reliable operation of intelligent systems in high-stakes environments such as water distribution networks, autonomous vehicles, and medical devices.