The SWIFT Project
The research in the Smart Water Infrastructure (SWIFT) project is directed towards optimization and control aspects of large-scale infrastructures with a particular focus on water distribution networks. To this end, we address various aspects of optimization such as multi-objective optimization, data-driven optimization using Artificial Intelligence (AI), robustness against system faults, cyber-attracts, and data privacy and security.
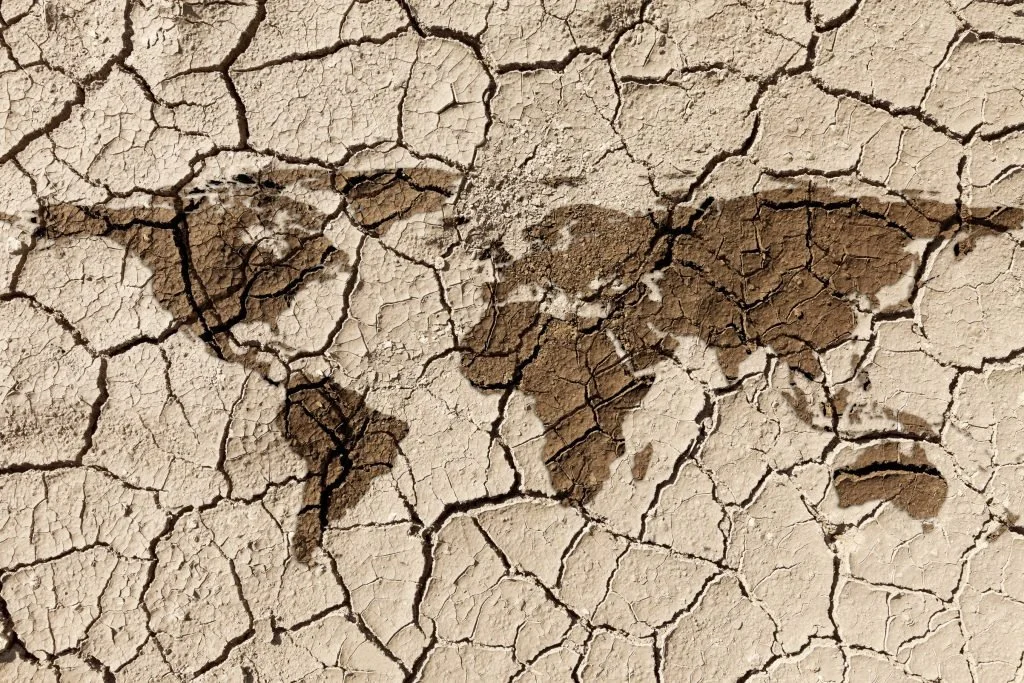
Have a chat with
RAHUL MISRA
about:
Decentralized control using game theory and RL
We have designed and implemented a decentralized control scheme using the notions of regret based reinforcement learning with guaranteed convergence to the optimal solution in the sense of correlated equilibrium. The designed solution is robust to disturbances due to variable consumption by consumers.
Have a chat with
SARUCH RATHORE
about:
Leakage diagnosis
• Detection, Localization, Identification.
• Our leakage diagnosis is based on pressure measurements.
• Estimated pressure residual signatures for different leakage scenarios are used to identify probable location of the leakages.
Have a chat with
JARON SKOVSTED GUNDERSEN
about:
Security and privacy in critical infrastructures
We live in a digital world, where many critical infrastructures are digitalized. We use different versions of encryption to ensure that private data is not compromised. However, we still want to be able to compute on the encrypted data. For this, we apply a technique called secure multiparty computation.
Have a chat with
SARUCH RATHORE
about:
Contamination impact mitigation
We have developed a control strategy for next-generation smart water networks. This strategy directs the flow of the contaminated water from a contamination source to a flushing point. This is achieved by creating a decreasing pressure gradient in the network through the regulation of consumer flows and supply pressure.
Have a chat with
ABHIJIT MAZUMDAR
about:
Safe Reinforcement Learning for Safety-Critical Systems
Maintaining safety is crucial during the operation of safety-critical systems. While reinforcement learning solves optimal control problems in a model-free setup, it does not ensure safety during the learning phase. Safe reinforcement learning circumvents this problem by embedding safety constraints into the learning process. Unlike conventional reinforcement learning, it uses safe actions more frequently during the exploration or learning phase. This method guarantees the reliable operation of intelligent systems in high-stakes environments such as water distribution networks, autonomous vehicles, and medical devices.
Have a chat with
Bulut Kuskonmaz
about:
Privacy Measurements for the Infrastructures
Privacy became one of the most crucial part in critical infrastructures. The algorithm used in critical infrastructures may compromise personal data of the users without consensual usage. To measure how much personal information the algorithm leaks the personal information, we can measure the amount of leaked data using the essentials of mutual information. We can use different metrics/divergences to have different interpretations of mutual information to widen our observation on leaked data.